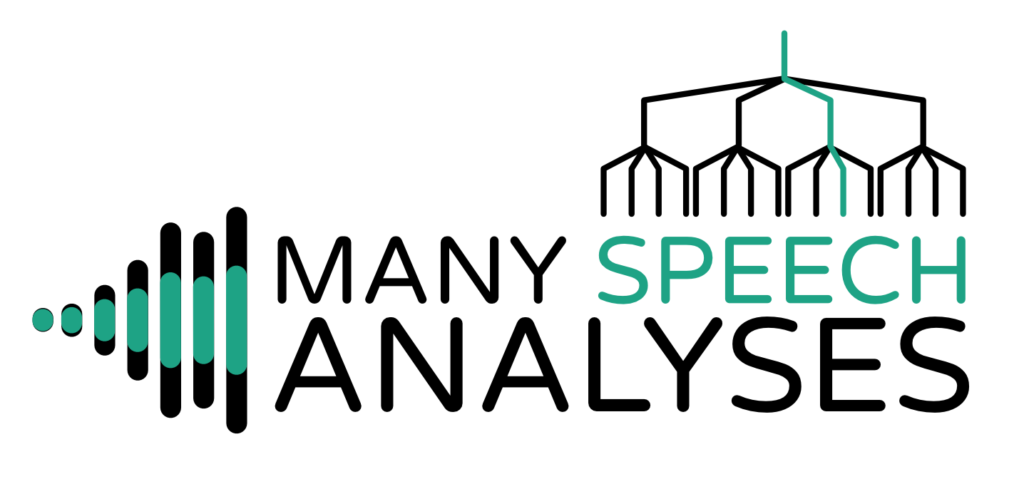
Help us improve speech science.
Be part of a large collaborative project.
We constantly do it, it is part of every empirical project. Data analysis. It’s a complex process. There are often many possible analytic strategies that could be used on one and the same dataset. This is true not only for the statistical analysis but also for the measurement itself. Speech scientists know that all too well. Comparing the acoustic signal of two utterances allows thousands of different analytical paths. What acoustic parameter do we measure? How do we measure it? In what part of the utterance do we measure it?
The Many Speech Analyses project tries to quantify this flexibility and explores how it affects our scientific conclusions. To that end, we are looking for speech researchers to analyze the same data set in order to answer the same research question. We are also looking for reviewers that will critically assess these analyses.
What is in it for you? If you agree on either analyzing the data according to our guidelines or review four analyses, you will become co-author of our paper. You will part of an international collaborative effort and help making speech sciences a more robust science.
THE DATA
Get to know the data set that we use for this project.
ROADMAP
Find out what happens next, if you sign up to be part of this project?
Blue Banana? The data set
Watch this short video
The dataset used in this project investigates the acoustics of referring expressions like “the blue banana”. Referring is one of the most basic uses of language. How does a speaker choose a referential expression when they want to refer to a specific object like a banana? The object’s context plays a large role in that choice. Generally, speakers aim to be as informative as possible to uniquely establish a referent (Grice 1975). Thus we expect them to only use for example a modifier like “blue” if it is strictly necessary for disambiguation (e.g. the adjective yellow when there is a yellow and a less ripe green banana).
But there is much evidence against strictly rational speakers. Speakers are often overinformative, i.e. they use referring expressions that are more specific than necessary. This redundancy has been argued to facilitate object identification and more efficient communication (Arts et al. 2011, Paraboni et al. 2007, Rubio-Fernandez 2016). For example, Degen et al. (2020) show that modifiers that are less typical for a given referent (e.g. a blue banana) are more likely to be used in an overinformative scenario (e.g. when there is just one banana).
The literature has a strong focus on whether a certain referential expression is chosen or not. However, speech communication much richer, allowing for more subtle enhancements of the communicative signal to make referential disambiguation easier. Spoken languages utilize suprasegmental aspects of speech to disambiguate referents and signal (un)predictable content. So we ask:
Do speakers acoustically modify utterances to signal atypical word combinations? (e.g. “a blue banana” vs. “a yellow banana”)?
To answer this question, we analyse recordings from an experimental study. We elicited sentences with noun-modifier pairs of varying typicality. Native German speakers were asked to instruct a confederate to select a specific referent among four objects presented on a screen. The instructions were presented to the subject via written form, which the subject read out loud to the confederate. An example input is “Please put the cube on top of the blue banana.”. The target noun-modifier pair was either typical (“yellow banana”), medium-typical (“green banana”), or atypical (“blue banana”). Your task will be to investigate whether typicality affects the acoustics of the pair.
Our road map
Project Coordination and Authorship
Stefano Coretta, Joseph Casillas and Timo Roettger, are the project coordinators and lead writers. They will receive the first, second and last author position, respectively. All other authors will be listed between the second and last author in alphabetical order. Contributors can earn authorship either as an analyst or as a reviewer. Being an analyst means completing and submitting a reproducible analysis within a stated time frame. This includes: (1) writing up a journal-ready methodology and results section, (2) answer a structured survey which gathers information on the analysis strategy and a description of the result, and (3) submitting the code for the analysis and a specification of analysis packages required to execute the analysis. Being a reviewer means completing and submitting reviews for four analyses reports.
Planned Timeline
There are five phases for this crowdsourcing project. In order to meet the timeline, some later phases may commence while earlier phases are in process. For example, parts of the report will be written while final data analyses are still under way.
PHASE 1 – Recruitment: Happening right now. Do you want to join our project? (Complete by 2021-##-##))
PHASE 2 – Analyses by analysis teams: Analyses conducted until 2021-##-##.
PHASE 3 – Reviews by expert reviewers: Reviews complete until 2021-##-##.
PHASE 4 – Analysis by project coordination according to submitted Registered Report.
PHASE 5 – Collaborative Writing: A paper draft written by the project coordination will be circulated to all authors (2021-##-##), asking for feedback (until 2021-##-##), before finishing write-up by project coordination (2021-##-##).
